
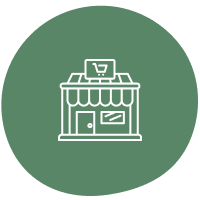
Secondary users
Extension-agents, Farm-retailers, -Farm-implement-manufacturers, Farm-implement-lessors/dealersPrimary target country
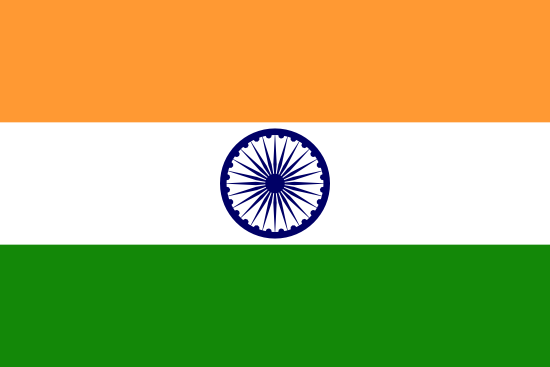
India
Through satellite and remote sensing, we are gathering ground reality of the aquaculture ponds. Active / Inactive ponds and Days of the culture.
Satellite estimates of inland water quality have the potential to vastly expand our ability to observe and monitor the dynamics of large water bodies. For almost 50 years, we have been able to remotely sense key water quality constituents like total suspended sediment, dissolved organic carbon, chlorophyll a, and Secchi disk depth. Nonetheless, remote sensing of water quality is poorly integrated into inland water sciences, in part due to a lack of publicly available training data and a perception that remote estimates are unreliable. Remote sensing models of water quality can be improved by training and validation on larger data sets of coincident field and satellite observations, here called matchups. To facilitate model development and deeper integration of remote sensing into inland water science, we have built AquaSat, the largest such matchup data set ever assembled. AquaSat contains more than 600,000 matchups, covering 1984–2019, of ground-based total suspended sediment, dissolved organic carbon, chlorophyll a, and SDDSecchi disk depth measurements paired with spectral reflectance from Landsat 5, 7, and 8 collected within ±1 day of each other. To build AquaSat, we developed open source tools in R and Python and applied them to existing public data sets covering the contiguous United States, including the Water Quality Portal, LAGOS-NE, and the Landsat archive. In addition to publishing the data set, we are also publishing our full code architecture to facilitate expanding and improving AquaSat. We anticipate that this work will help make remote sensing of inland water accessible to more hydrologists, ecologists, and limnologists while facilitating novel data-driven approaches to monitoring and understanding critical water resources at large spatiotemporal scales.